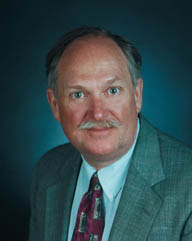
Many variables go into determining a batch's rank and typically a limited number of people are able to observe the cause and effect of more than a few variables. If we look at the production process as a black box, we can see a series of ingredients, each with a set of measurable quality parameters. For example, if an ingredient is a fruit juice, the quality parameters may be acidity, percent of solids, brix, etc. We also see a series of process variables (temperature, pressure, etc.) that impact the ranking of a batch.
To understand the relationship between these variables and batch quality, we need to look at a series of batches, tracking and comparing the data from each. Understanding the correlation between batch ranking and these variables enables us to create the conditions that produce a desirable outcome and improve the batches.
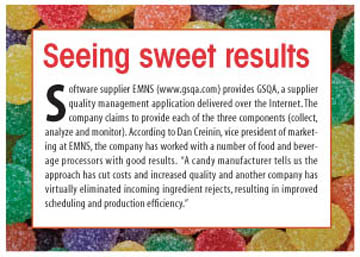
The information on individual ingredient lots and end products is typically available but not in a format that we can use directly. Ingredient parameters may come from the suppliers Certificate of Analysis (COA), your own test labs or outside testing facilities. Process parameters may be available from data historians, MES or other control systems. Finished product results will come from testing.
In order to improve, the existing information has to be made available from various existing sources to an application that can perform the analyses. The more data you have, the better the results. However, even limited data may help you improve.
Second, we need to analyze the information and look for the correlations between the input parameters and batch ranking. Various statistical processes yield information that shows us the common elements that improve profit potential.
Analysis may reveal that some variables actually have limited impact on the quality of the batch. For these non-impacting variables, you may be able to move to a lower quality ingredient to either reduce cost or change the range of acceptability. In other cases, you may find a direct correlation between ingredient quality and batch quality. You may want to minimize the range of acceptable ingredient quality in order to create better batches.
Is this analysis a one-time approach to improvement? No, the approach works on an on-going basis, contributing to continual improvements and improved profitability. By consistently identifying the cause and effect behind terrible batches, you can lift every batch's ranking.
Olin Thompson is a consultant who specializes in the application of information technology in the food industry. He is the founder of The Food CIO Forum (www.foodcioforum.com).