Tech Update: Taking Control

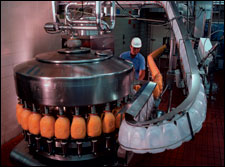
Hence, many processors are implementing more advanced systems, known as predictive and adaptive controls, to regulate these processes. Most such systems base control decisions on comparisons between mathematical models of the process and the process itself, thus allowing the controller to predict where a process is heading; identify the control efforts required to keep the process within specifications; and make the adjustments required to ensure that specifications are maintained. Continual input/output data from the process are used to update the model while it operates, so that it can account for process changes and continue to optimize performance. Assuming, for instance, that production rates change, the predictive adaptive controller in turn modifies response time.
Depending on the supplier, controls are based on theoretical models or, in the case of United Dynamics Technologies' BrainWave product, the actual performance/actions of the process. According to the supplier, BrainWave builds its own mathematical model of the process by observing how process signals relate to each other and then uses the model to make accurate forecasts of the process response.
The BrainWave product simply requires an estimate of process dead time and the time constant. Once this information is provided, models are built from the actual process, with the controller using the models to predict what the control variable should be. According to the supplier, the models can adapt to set point changes or, in the case of feedforwards, adapt continuously. BrainWave has been used for a variety of food and beverage applications, including pasteurization, kettle control, pH control, dryers, heat exchangers and boiler control.
One recent application involved batch processes for the production of emulsifiers, monoglycerides and other ingredients at the Williamsport, Tenn., plant of manufacturer Lonza, Inc. According to Lonza process engineer Joshua Mensinger, who made a presentation on the application at last June's PlantTech conference, the process was nearly impossible to control with conventional PID loops since reactions involved long dead times and dynamic changes as batch mass increased. Consequently, existing PID loops were replaced with a BrainWave control system capable of modeling and adapting to dead time, learning the process, and continuously creating feed-forward models that adapted to changing dynamics, Mensinger explained.
At the Lonza facility, BrainWave runs on a Windows-NT PC integrated with the existing Rosemount R3 distributed process control system via an OPC server. While initial models were developed from historical data, BrainWave was shifted to automatic mode and completed an entire batch on its first run. Mensinger said that temperature swings have since been reduced from 20 to 5 degrees C. Flow rate has increased by 30 percent, while reaction times have declined by 20 percent. Payback, he said, was achieved in four to five months.
Juice-maker Ocean Spray Cranberries employed BrainWave to achieve greater control of the pasteurization process at a Henderson, Nev., plant that blends and bottles cranberry juice, among other beverages. Juice concentrate at the plant is sent through a balance tank that combines it with recirculated juice to feed the first zone of the pasteurizer, which additionally features a second zone, a hold loop and a trim cooler. Steam supplies heat to the two zones to raise juice temperature for pasteurization, after which the juice moves to bottling and off-spec product is recirculated to the balance tank.
With daytime temperatures in Henderson rising to 100 degrees F. or greater during the summer, Ocean Spray had difficulty controlling the temperature of process water in the trim cooler used for adjusting the temperature of juice as it was being bottled. Similar problems arose with process water for chilling recirculated juice before it returned to the balance tank, resulting in erratically cooled juice recirculating through the pasteurizer. (FDA regulations specified that the juice remain within +/- 1 degree F. for a specified time.)
During the summer, the plant's original PID controllers could take as long as 30 minutes to stabilize the pasteurizer's temperature start-up. By implementing Brainwave, which runs on a PC and connects serially to a PLC, Ocean Spray was able to achieve tighter real time control of the pasteurizer. Start-up time was reduced by 70 percent as a result of a feedforward scheme that anticipated incoming fluctuations in juice temperature, allowing the controller to make corrections before the pasteurization process was adversely affected.
According to Brainwave product manager Bill Gough, United Dynamics plans to release a new multi-variable version of the product next year featuring multiple interacting loops. "Whereas our current product allows multiple inputs but only a single output, the multi-variable version can generate several outputs," Gough said, acknowledging that the multi-variable product may exceed the requirements of many advanced control applications in the food industry.
Adaptive Resources' QuickStudy [likewise] generates process models from either historical or real-time operating data [without bump testing], using the models to predict the direction a process is taking and make adjustments before the process can deviate from setpoints. The models can be continually updated to meet changing process dynamics and predict the effects of control changes and feedforward events, thus allowing the process reach setpoints quickly and without oscillation, even in the case of long time delays or variable dynamics.
A few years ago, Adaptive Resources released a modeling and simulation tool that develops process models off-line from a few days of historical data. Named Q-MAST, the program can model complex processes with highly interactive variables, long dead times - integrating characteristics and inverse responses without any plant disruptions or response testing.
As reported last summer in Food Engineering, a QuickStudy Adaptive Process Controller (APC) developed by Adaptive Resources was installed for testing on a Thermally Accelerated Short Time Evaporator (TASTE) at a Florida orange-juice plant. Using evaporation to concentrate juice for storage and transportation, the plant also wanted a control system that more tightly regulated the final Brix of the concentrated juice. To do so, operators took several hundred data points and used Q-MAST to create the model offline, then installed the model in the system, let it run and evaluated it, updating it with new data as the process was running. Several variables were taken into account during modeling, including feed rate, steam pressure change and feedstock concentration. While a conventional process typically controls variability in the final concentration to plus or minus 1 degree Brix, operators were able to control variability to within 0.1 degree Brix over any two-hour period.
Pavilion Technologies' Process Perfecter, a multivariable model predictive control program, has been used in numerous closed loop control projects involving milk evaporation and drying, perhaps most notably for Anchor Products Ltd., the manufacturing arm of the New Zealand Dairy Group. Process Perfecter employs process or simulator data to build steady-state models, which are in turn used to help identify a dynamic model for the process. The two models are then combined into a unified, consistent controller, according to the manufacturer. New knowledge is continuously applied to the model in real time. The learning process is continuous rather than intermittent, facilitating steady-state optimization of the process.
Version 6.0 of Pavilion's software suite, released last September, introduces a new data visualization feature that includes 3-dimensional model analysis plots and the ability to selectively overlay variable plots. It also provides more robust data screening tools and a configuration assistant, Perfecter Builder, that allow operators to configure and modify on-line Process Perfecter applications without disturbing the process.
Although the vast majority of adaptive and predictive controls are model-based, at least one, the software system CyboCon, from CyboSoft, a unit of General Cybernation Group, Inc., is not. Unlike model-based systems, the CyboCon product doesn't require any process identification, quantitative process knowledge or controller design. Nor does it require complicated manual tuning, as PID loops do.
While the original CyboCon product runs on a PC interfaced to a PLC or DCS, a more recent version, CyboCon CE, runs under Windows CE on a dedicated control box.
Until recently, the company has played its cards pretty close to its vest, offering little explanation as to how the software manages to provide effective feedback control with no model of the process and only nominal operator intervention. But having finally patented the technology, CyboSoft president Dr. George Cheng shared some details of the program with the European edition of Control Engineering magazine earlier this year. According to the account, the software looks at the error between setpoint and process variable to determine how to manipulate the process, much as any feedback controller does. However, CyboSoft analyzes a whole string of error measurements recorded during the previous N sampling intervals, allowing it to observe the dynamic behavior of the process. Rather than create a fixed model from these observations, CyboSoft employs a dynamic neural network to compute the next control effort directly from the error history. By continuously updating the values of weighting factors, which allow the network to recognize or learn patterns embedded in a set of measurements, the network repeatedly helps generate adaptive control signals at the end of the sampling interval in order to minimize the error between setpoint and process variable.
In a recent phone interview, Cheng indicated that one of the principle advantages of the model-free approach is ease of operation. "It would be relatively easy for an engineer with basic training in controllers to launch our product," Cheng said. "Model-based controllers typically require greater expertise on the part of their operators."
Looking for a reprint of this article?
From high-res PDFs to custom plaques, order your copy today!