Maintenance strategies are often categorized as reactive (wait until it breaks), preventive maintenance (PM), reliability centered maintenance (RCM), risk-based PM, condition-based monitoring—otherwise known as predictive maintenance (PdM)—and prescriptive maintenance, which uses analytics—such as AI—to indicate equipment is headed for trouble, and can prescribe and prioritize mitigation and/or repair).
You may think you have a well-defined maintenance strategy in place, which for example, uses sensors to track bearing temperatures on a critical 200 hp motor and has operators informed on what to do when bearing temperatures rise. But a bad decision in taking action can do more than shut down a line; it could be disastrous. A good example is last winter’s Norfolk Southern freight train derailment with a hazardous chemicals spill and resulting fire in East Palestine Ohio. And you can’t say they didn’t have data.
Even with track temperature sensors in place and spaced 11 miles apart, Norfolk Southern failed to slow down and stop the train before the 23rd car of the 149-car train experienced a sharp rise in wheel bearing temperature to more than 250°F above the ambient outdoor temperature of 10°F. According to an NPR report, the initial findings of the NTSB showed that the first track sensor to indicate a temperature rise recorded a temperature 38°F above the ambient; a second sensor farther east, 103°F; and a third sensor, 253°F.
Train company guidelines suggest a take-action point when wheel bearings reach 170°F above ambient. Once a temperature of 200°F is reached, operators are required to stop the train immediately and remove the car. Unfortunately, by the time remote dispatchers issued an E-stop to the train’s engineer, the bearings on that car had begun to seize, creating an uneven stop and derailment—and resulting fire. One has to ask, though armed with the rising temperature sensor data, why didn’t the railroad see a trend developing that could have resulted in an early warning to slow and stop the train gently before bearings seized on the 23rd car?
Factors That Can Hinder Good Maintenance Strategies
As with the railroad, food and beverage processors may have the beginnings of a good maintenance strategy in place, but several issues can complicate the administration of it. For example, here’s a quick dozen:
- Poor equipment reliability and downtime reduction
- Aging infrastructure
- Talent shortage and skills gap
- Technology adoption is slow
- Robust data management and analytics is needed
- Budget constraints inhibit good maintenance strategies
- Regulatory compliance and machine safety/food safety
- Sustainability and energy efficiency
- Supplier relationships and spare parts management
- Communication and collaboration
- Poor change management and control
- Lack of remote monitoring capabilities
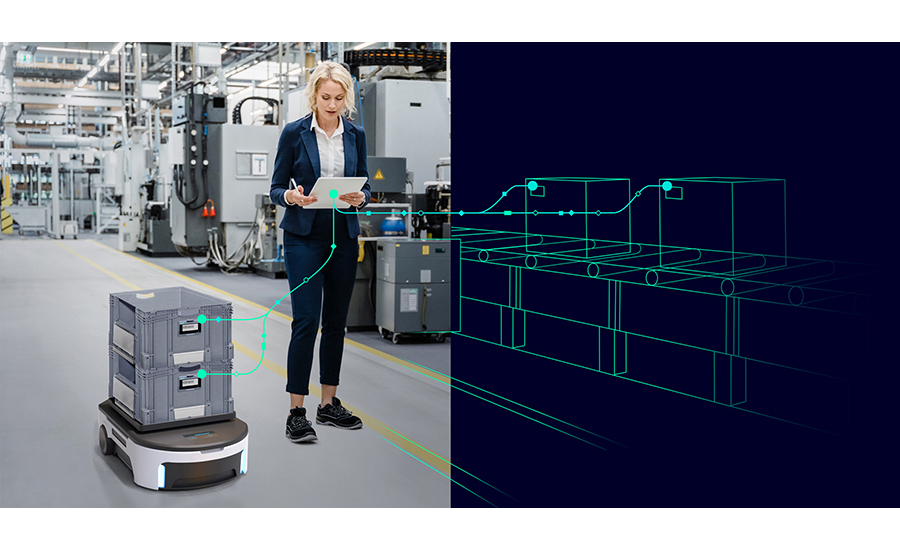
Strategizing Maintenance around Major Issues
What do maintenance providers, engineering houses and sensor manufacturers see as the main stumbling blocks/needs in the above list? Corey Cronin, director of business development, food & beverage | packaging at Emerson’s Discrete Automation Group, lists three. Equipment reliability and downtime reduction, asset utilization, and OEE are critical KPIs driving maintenance managers’ efforts to get as much out of their equipment as possible, making sure it is as reliable and efficient. “Sustainability and energy efficiency are an equally important driver and concern. Many of our customers are particularly focused on reducing plant air consumption, which in some facilities can be up to 30% of the total energy used in the facility. They are investing in point-level power monitoring and air monitoring systems that provide real-time data to drive preventive maintenance practices and efforts to eliminate pneumatic leaks in as many areas as possible.”
Cronin also notes that talent shortage and loss of skilled operators are food processors’ number-three concern, and there has been increased interest in improved SCADA platforms and machine data reporting tools so processors have the information to respond to downtime activities and can better prepare for and plan maintenance strategies.
“The most pressing maintenance issues I see food and beverage clients face are equipment reliability and downtime reduction, aging infrastructure, and talent shortage and skills gap,” says David Ziskind, Stantec market lead, food & beverage. “Ready, willing and able labor talent is important (skilled and available). Infrastructure may be aging or on a long capital replacement cycle. On the positive side, I see more clients dig deep from the sustainability and energy efficiency angle when replacing equipment, although I don’t often see equipment replaced prematurely to capture this, unless it offers a very compelling ROI.”
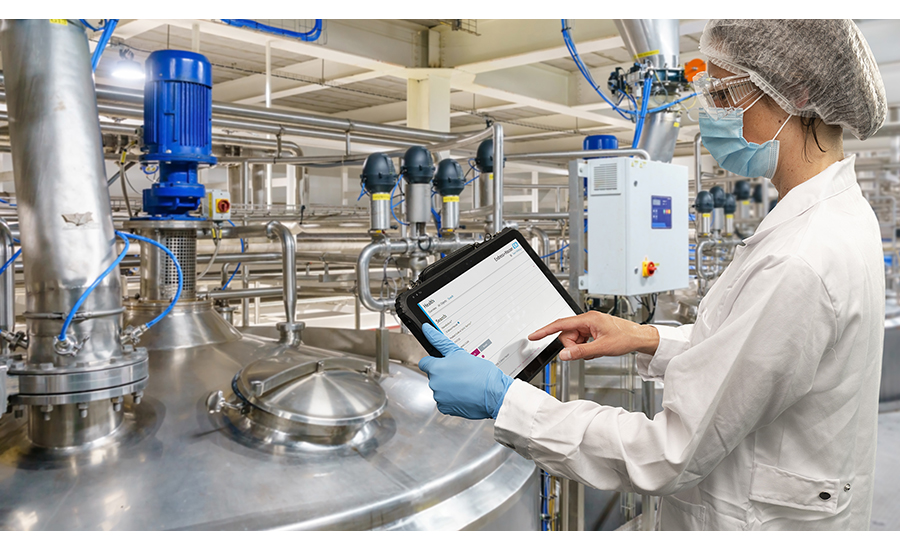
Though not directly stated on the list, calibration and documentation activities are major concerns for processors, says Jason Pennington, Endress+Hauser USA director of digital solutions. “More frequently, however, these discussions are trending toward installed base analysis, standardization and digitalization strategy co-development. We are in a time void of a straightforward approach. However, there are core themes such as empowering technicians and working toward a more predictive future [plus] overall continuous improvement through data analytics—all of which are leading initiatives and projects.”
Modern technology adoption is a key issue that goes hand-in-hand with talent shortage and the skills gap, says Gregory Perry CMRP, CRL, eCMP, Fluke Reliability senior capacity assurance consultant. As technology becomes more human centric, it is inevitable that it marries with the asset centric realms. Not to be seen as a replacement for talent shortage, artificial intelligence, machine learning and edge computing are steadfast to augment the skills gap. Merging the best of technology with the best of human capital is a win-win, Perry adds.
Erin Sidwell, Fluke Reliability key account manager, food and beverage/process manufacturing, chose equipment reliability and downtime reduction, talent shortage and skills gap, and data management as three top concerns. “Food and beverage companies look for solutions that simplify food safety and regulatory compliance [and] keep production lines moving. Digitization of work orders, standardization across the organization and automation push to end unplanned downtime and sustainably meet customer demand.”
Replacement parts in equipment and pumps that come in contact with food must meet FDA’s CFR 21 Part 77 regulations. Pump and pump parts suppliers, like Anderson Dahlen (A Gray Company), work with food companies to ensure that the correct parts are ordered and shipped, according to Kris Rarden, business development manager.
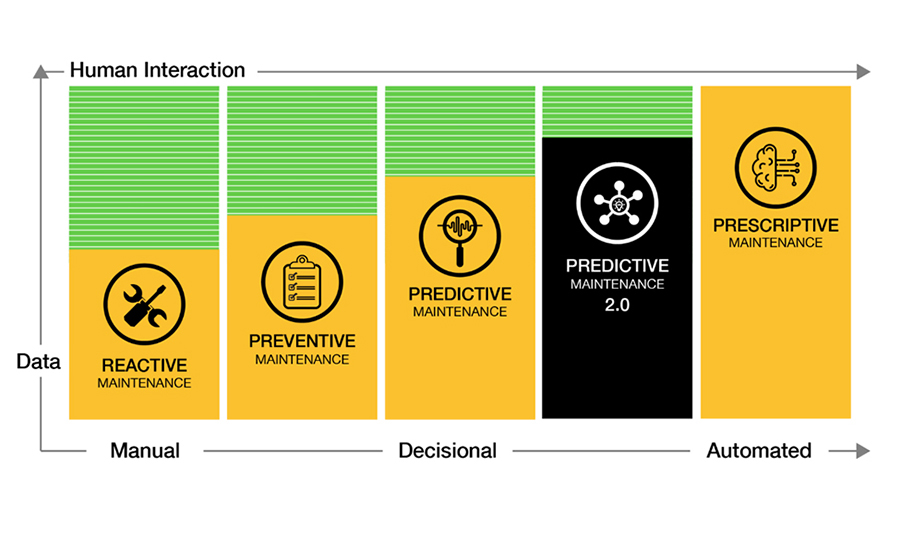
Two items on the list create tricky problems for processors, according to Jeremy Reed, manager, instrumentation, Gray Solutions, A Gray Company. Aging infrastructure and equipment and reliability/downtime reduction are two areas that often present related issues—as new technology emerges, some of the older software and hardware can become scarce, expensive, or even obsolete. The processor can’t pivot overnight so a lot of these older components are required to keep running. This means more wear and tear along with less downtime for preventative maintenance. Paying special attention to maintenance metrics such as MTTF (mean time to failure) in these situations can help end users understand the limits of their systems and better determine schedules for maintenance. Over-running or under-maintaining can turn into an exercise in diminishing returns—especially when the parts in question are part of an aging infrastructure.
But aging infrastructure isn’t just equipment like large motors or HVAC systems. “Interestingly, it’s the aging infrastructure of our customers’ buildings that often creates the greatest challenge,” says Brad Moore, principal of reliability at Pinnacle Reliability. “For example, one of our customers experienced a leak in the roof of one of their buildings, forcing the facility to stop production.”
Data and Retaining Employees—Related Issues That Go Hand-In-Hand
Moore continues, “This same customer, along with many others in the industry, also struggles to retain employees with extensive subject matter expertise. It’s common for facilities to not have organized data management systems in place, which makes it even more challenging when an employee and their unique knowledge of how the facility operates leaves.”
“We see many food and beverage facilities struggle with not having enough data, staff or resources to complete necessary work,” says Pinnacle senior engineer Bruce Copple. “One reason this can occur is when a facility does not properly conduct a root cause analysis (RCA) after a failure. As a result, these facilities often experience repeat failures, which can add to the amount of work and lead to reactive maintenance practices.”
One way facilities can address this challenge is by conducting a failure modes and effects analysis (FMEA), says Copple. In addition to identifying the functional requirements of an asset or system, an FMEA provides documentation that can also be used to train new people on how a specific piece of equipment can fail. This analysis captures critical asset information and can be passed down to the next generation of employees, alleviating some of the stress that can come with high turnover.
“Another common challenge we see with our customers is a lack of trust in their data,” adds Moore. “This lack of trust often stems from having poor-quality data (e.g., missing data or conflicting data). For example, one of our customers completes many preventive maintenance (PM) work orders outside of the centralized maintenance management system, and as a result, different groups do not have access to the complete set of information. Some operators will say that they’ve never seen a specific piece of equipment break down, while others will say the exact same piece of equipment is not reliable. Ultimately, the root of their challenges is data quality and analysis.”
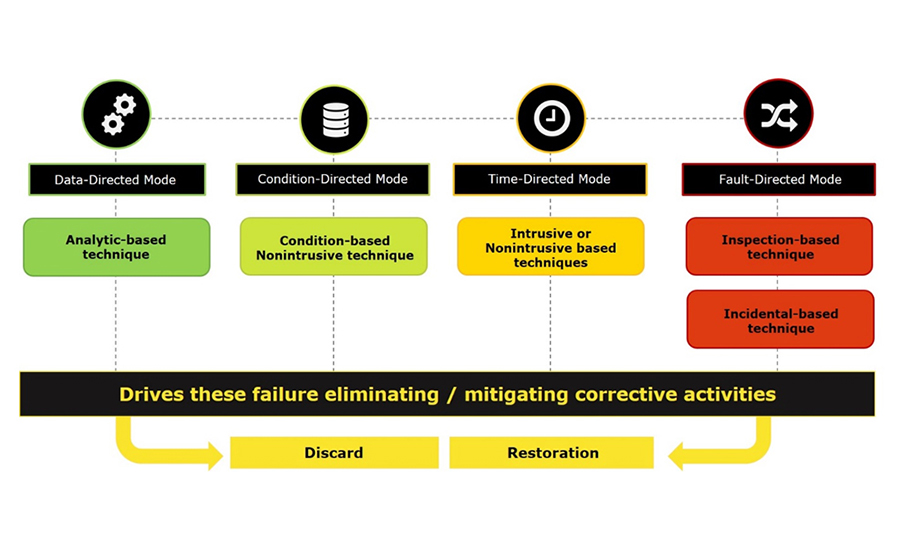
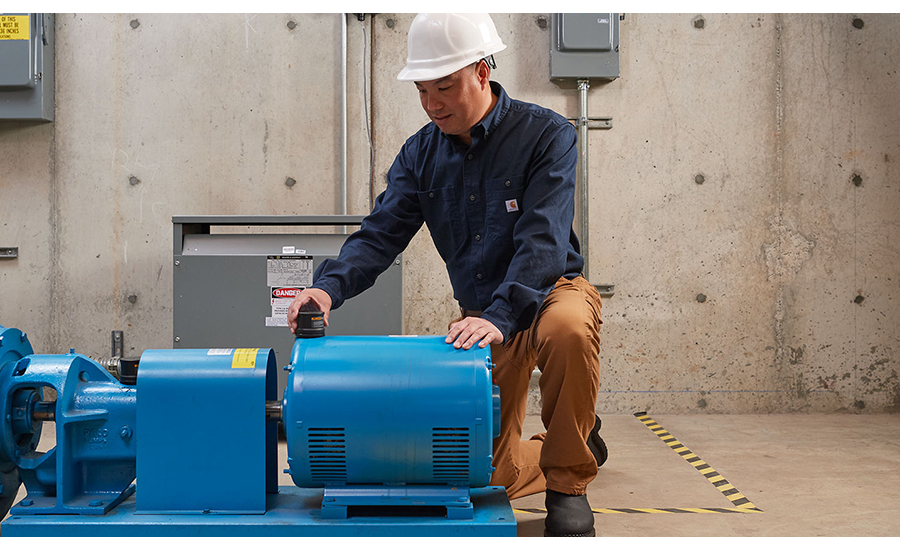
“Checking for problems that don’t exist and maintaining assets that don’t require it is a waste of time, especially given limited labor resources in today’s tight employee market,” says David Peterson, Siemens solutions engineer, Senseye Predictive Maintenance. “PdM helps facilities leverage their limited personnel resources more efficiently by calling attention to machines and other assets showing signs of reduced health.”
Digital tools like PdM help capture best practices and tribal knowledge in all manufacturing areas, especially in maintenance, says Richard Phillips, director of smart manufacturing, Polytron, Inc. “A maintenance management system can enable training for new employees by delivering standard operation procedures (SOPs) when and where needed.”
Speaking of new employees, don’t overlook younger people. “Younger generation employees are not only accustomed to using digital tools, but also expect them in their workplace,” adds Phillips. “Giving your workforce the digital tools they need to be productive creates a culture of continuous improvement.”
For example, manually monitoring hundreds or thousands of assets in a facility is extremely time-consuming, says Peterson. Automated condition monitoring software, such as Siemens’ Senseye Predictive Maintenance, reduces this burden, providing constant asset health data and alerts when attention is required. This frees up plant personnel’s valuable time and facilitates a more proactive approach to maintenance.
Prevent a Train Wreck—Move Away From Reactive Maintenance!
In many cases, processors may remain in a reactive state, but not for technology reasons. “One way that our customers can get to a more proactive maintenance program is by establishing good procedures for closing out work orders and capturing valuable data,” says Pinnacle’s Copple. This usually involves using pick lists in mandatory fields. Additionally, these facilities need to ensure that their employees are trained on these procedures so all groups are collecting, monitoring, and auditing quality data.
In my own past experience, quality data means describing a problem better than “machine broken” or “transmitter doesn’t transmit.” What part of the machine is broken? How did it break? Did someone check the transmitter fuse? What are the visual indications?
As Pinnacle’s Moore suggests, completing PMs only to check a box that says “repaired machine” leaves little in the way of useful information, certainly not enough data to digitize in order to shift to a more proactive maintenance system.
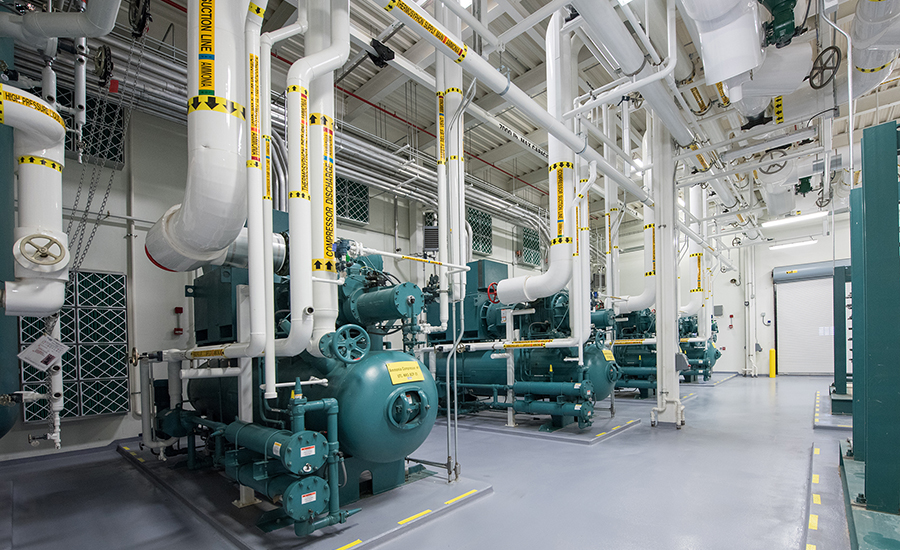
Many of Emerson’s clients’ maintenance strategies are evolving from reactive to preventive maintenance programs, investing in better planning and processes to stay ahead of equipment breakdowns and inefficient performance and drive improvements in OEE, says Cronin. “What many aspire to achieve is what we refer to as autonomous maintenance, which is part of reliability-centered maintenance, or RCM.
“We can make RCM a realistic methodology by having better data and better technology for gathering and integrating the performance data from across machines and production lines,” adds Cronin. Key indicators that forecast potential problem areas are critical: Are devices or lines operating out of spec? Are we seeing excessive runtime on a device? Is a drive pulling more power than normal, and is that an indicator that some physical component like a bearing is undergoing excessive wear and needs replacement? By getting more information from our machines, the maintenance managers can adjust their PM programs much more effectively.
Moving From PM to Risk-based PM and PdM
The maintenance management solutions that Polytron is deploying typically include PM and PdM, says Phillips. The solution allows scheduling and managing PMs based on machine runtime, schedule availability and many other factors. PdM using vibration sensors and baseline analysis is added for assets that would cause significant downtime if they were to break. The PdM data is fed into the MES platform in order to automatically generate work orders.
Many manufacturers, however, are driven to improve maintenance but still struggle to understand what the best practices are and best technologies they should be using to develop their strategy, adds Phillips.
This struggle is often compounded because they have older, legacy machinery mixed with newer edge devices that they’re trying to integrate over fragmented networks ranging from Ethernet to LoRaWAN (long-range radio) to cellular networks, says Siemens’ Peterson. Some OEM equipment might come with sensors already installed, but others are bare and do not natively generate much health data. Even those that do produce health data are rarely understood by a typical maintenance professional.
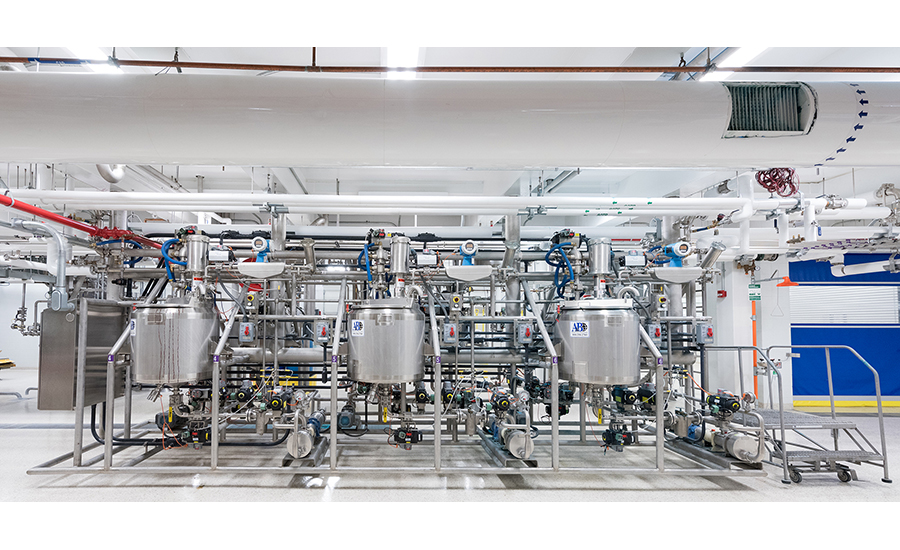
Adding to the confusion is a bevy of disparate software applications that only communicate with select sensors or controllers, adds Peterson. Most Siemens food and beverage clients are in the PM stage, however the majority aspire to make the digital jump to PdM. Because Senseye Predictive Maintenance is sensor, network, controller and infrastructure agnostic, users can seamlessly combine legacy equipment with edge devices—regardless of vendor.
Predictive and prescriptive maintenance are the industry goals, says Endress+Hauser’s Pennington. It’s relatively easy to align these goals with overarching issues surrounding labor, productivity gains and cost avoidance. However, one of the largest challenges remains the IT/OT divide. Many processors acknowledge that good data and information is trapped in their assets and legacy control systems, which were installed well before digital strategies were in place.
Most processors who are Gray Solutions clients operate with some level of PM programs, says Matt Meiser, manager, digital transformation. Many aspire to operate at the prescriptive level but lack the infrastructure and contextualized data required to do so at existing facilities.
Fluke’s Perry suggests that some food processors—that while not necessarily in the reactive mode—may be held back to risk-based maintenance because of the type of industry. “Being a heavily regulated industry such as food and beverage, second to pharma, risk-based maintenance strategies are more prevalent. In these approaches, companies are held more to OEM limited maintenance-and-repair approaches. That is where technology can become an augmented resource of value.”
Risk-based maintenance or risk-based PM prioritizes maintenance resources toward assets that carry the most risk if they were to fail. It is a methodology for determining the most economical use of maintenance resources. “Risk-based PM—many of our clients prioritize calibration and audits of their most critical measuring devices and operational equipment,” says Adam Dittbenner, instrumentation manager, Interstates, a CSIA certified member
PdM is at the top of digitalization efforts within food and beverage, and adoption is rising, says Fluke’s Sidwell. According to one processor, plants are still reacting to the assets more than 60% of the time and want to shift to a predictive model to save costs and maximize uptime. PdM plays a vital role in sustainability by increasing operational efficiency and preventing issues before they cause environmental damage. Additionally, PdM helps manage energy consumption by rectifying energy-intensive equipment early, saving maintenance resources and costs, and keeping workers safe.
To the Edge and Beyond…
“Some of the most valuable and effective tools available to move to next-level maintenance strategies are the new generation of edge computing systems,” says Emerson’s Cronin.
While “edge servers” provide the connection between a SCADA or MES system and the cloud, the main concept when applied to maintenance is to collect the data continuously (e.g., vibration, temperature, overcurrent) from assets such as motors, grinders, etc., and move it to the cloud and let the heavy-lifting of crunching that data with AI/ML to somebody else. Once processed, the “cloud” system can then let you know which equipment needs serious maintenance evaluation. Thus, your maintenance system is not necessarily burdened with collecting mountains of maintenance data. Instead, an edge server relays it to the cloud for processing—you simply pay a monthly bill.
“Many of our customers already have the foundation and a process historian, but the implementation has often been an afterthought,” says Gray Solutions’ Meiser. Ensuring that that the facility is well modeled to add context to the data makes it possible to take the next step of making data available to advanced analysis and AI/ML tools. In addition to offerings from traditional vendors in the manufacturing space, cloud vendors are starting to make tools available for the manufacturing space. One example is Amazon Lookout for Equipment.
Systems such as Emerson’s PACEdge controller platform are integrating machine learning and AI capabilities into these systems so they can simultaneously be a controller for a packaging or food processing machine while independently functioning as a powerful data collection and analytical tool to monitor performance of devices across a machine and guide smarter, real-time preventive maintenance.
There are AI/ML tools that can run or be deployed at the edge as inference-based models, or in the cloud as real-time computer cycles. “One of Endress+Hauser’s greatest opportunities is leveraging our 70 years of device data, knowledgebase of troubleshooting and unique ability to work in parallel with the control system to provide labels or fact-based insights,” says Pennington. “For example, many systems are available that can help identify anomalies. It’s the ‘what’s next?’ or ‘here are remedies based on that anomaly’—forward-looking approaches, which are extremely meaningful to our customers—that we are working on improving.”
In addition to cloud options, many maintenance and reliability companies have teamed up or purchased companies with AI/ML expertise. “The recently announced Azima DLI acquisition further accelerates the shift toward AI-enabled predictive maintenance for Fluke Reliability customers,” says Sidwell. “With today’s skilled labor shortages, even teams with existing condition monitoring programs often struggle to analyze their data and turn it into action. Azima DLI’s advanced diagnostic engine analyzes about 93% of machine tests without human intervention.”
The good news is that AI/ML and cloud technologies don’t have to stop with PdM systems. Says Polytron’s Phillips, “Once these systems are installed, AI/ML can be used on the platform data to uncover key insights not only within maintenance, but across all MES functions.”